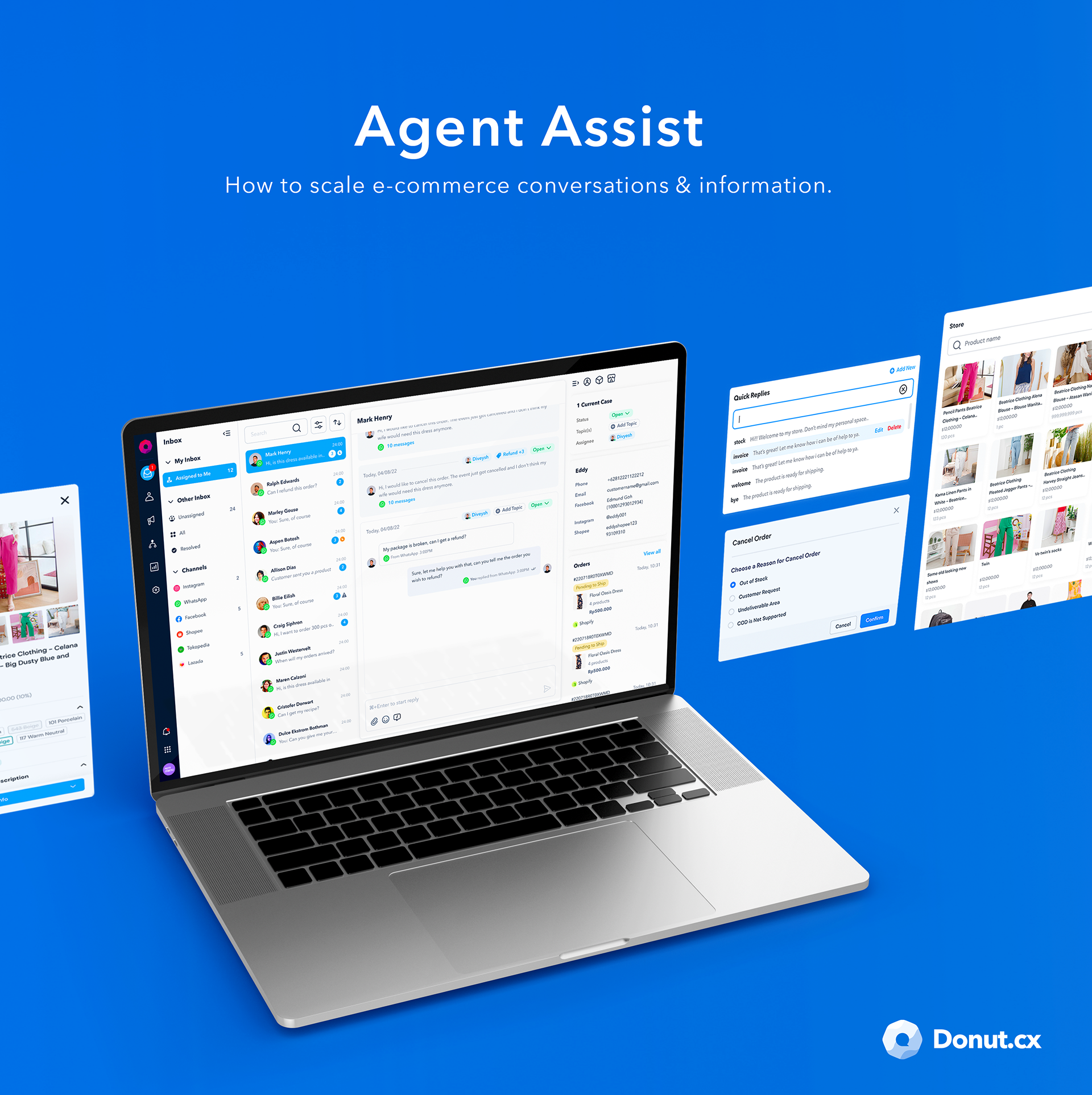
Agent Assist
Donut.cx, an e-commerce platform, pivots to find product market fit in empowered chat aggregation.
Summary
Using Miro, Figma, and every Research & Brainstorming tool out there, we created an e-commerce conversational support and empowerment platform, fueled by 'next-best-action' features like: canned responses, automated intent-based topic tagging, on-demand product data, etc.
Problem
For over two years, Donut.cx (formerly LummoSHOP) offered an e-commerce marketplace management SaaS solution catering to small to medium-sized businesses in Southeast Asia. However, they've still met challenges in finding the right market fit.
After conducting extensive research on our customer base, we identified a common pain point shared by all our business partners: the challenge of effectively managing customer conversations and relationships across multiple platforms and channels. This burden often translates to customer service representatives handling an overwhelming volume of inquiries, sometimes exceeding a hundred interactions per day, while also expecting them to address and resolve complex technical issues.
Phase 1 - Research
A - Initial Interviews
From the outset, we prioritized qualitative research to gain valuable insights. Through activities such as day-in-the-life studies, card sorting exercises, and even journaling, we diligently mapped each user profile, uncovering their unique agendas and challenges.
Essentially, we were solving for the age-old problem of people becoming overwhelmed; being in dire need of improved visibility, and accessibility. Note: the data gathered here highly influenced the eventual user archetypes, and JTBD's.
B - Competitive Benchmarking
Undertaking an extensive competitive benchmarking analysis, we meticulously identified the leading products that demonstrated exceptional performance in verticals closely aligned with our target market. Drawing upon their expertise, we diligently documented their design patterns, business strategies, feature sets, and even their market penetration. Through this exercise we unravelled the factors and features contributing to competitors outstanding achievements and favorable user reviews.
These features/advantages are then synthesized into manageable groups, or convictions. Dedicated teams then focused on productizing the insights--MVT groups.
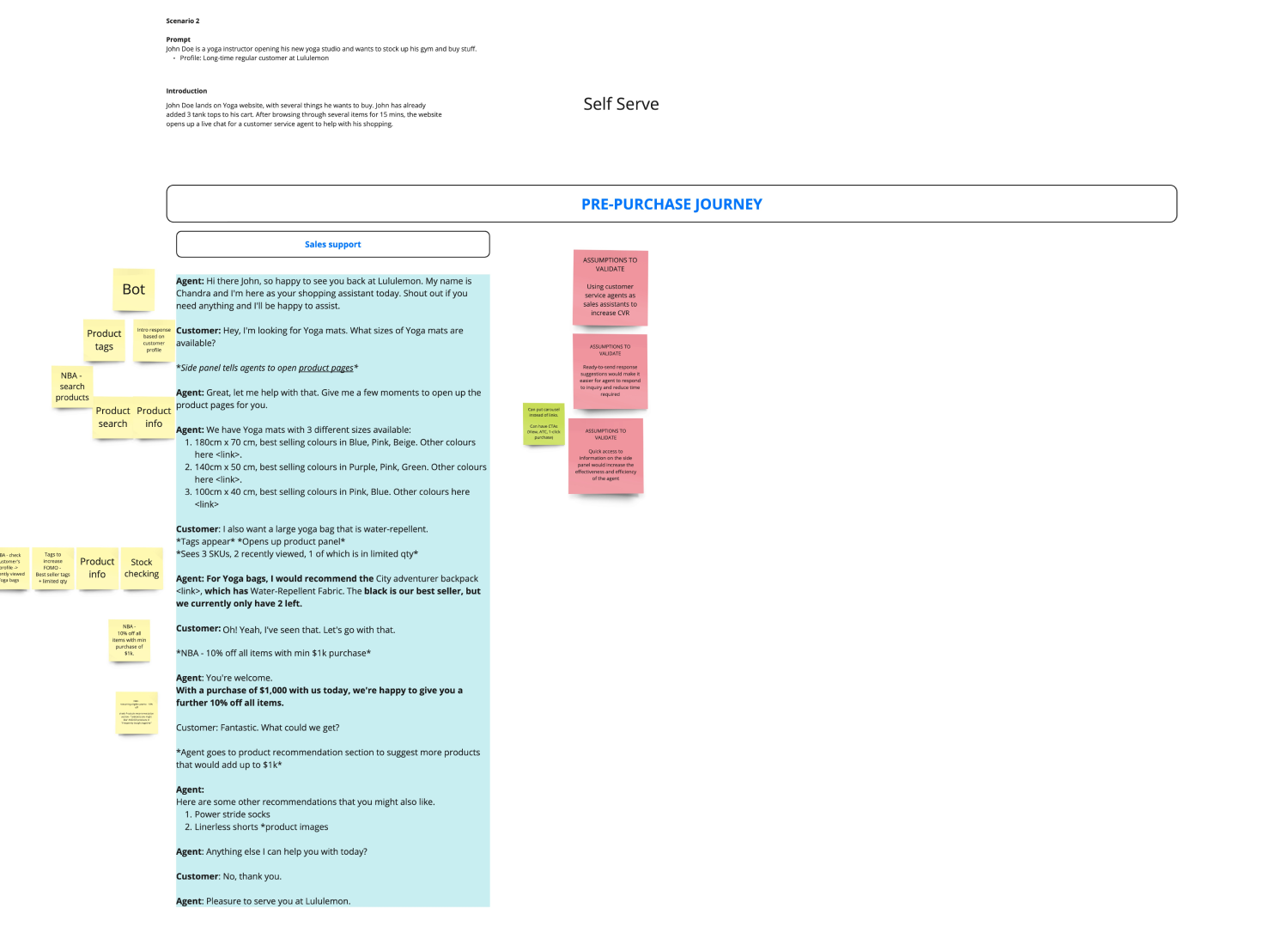
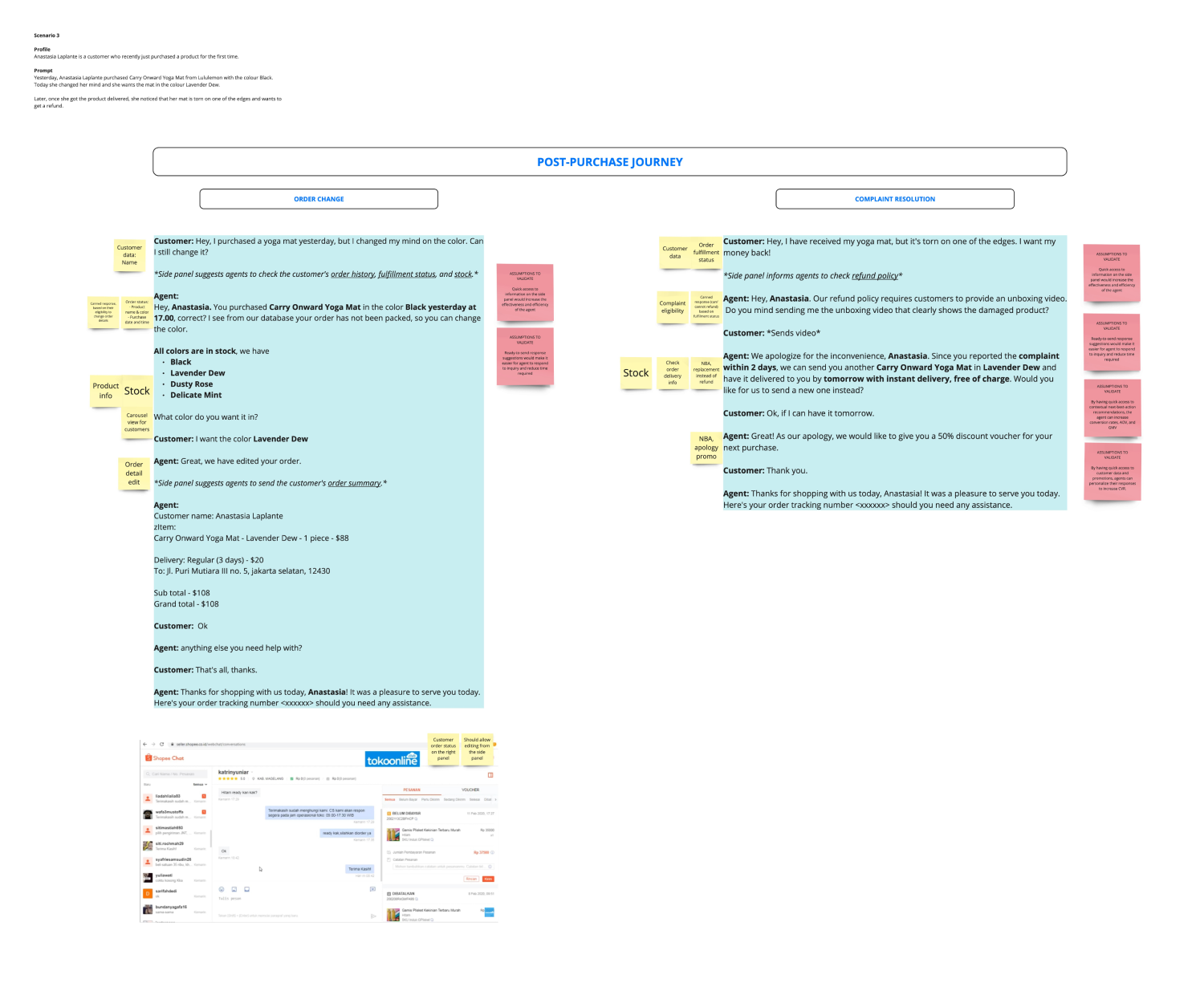
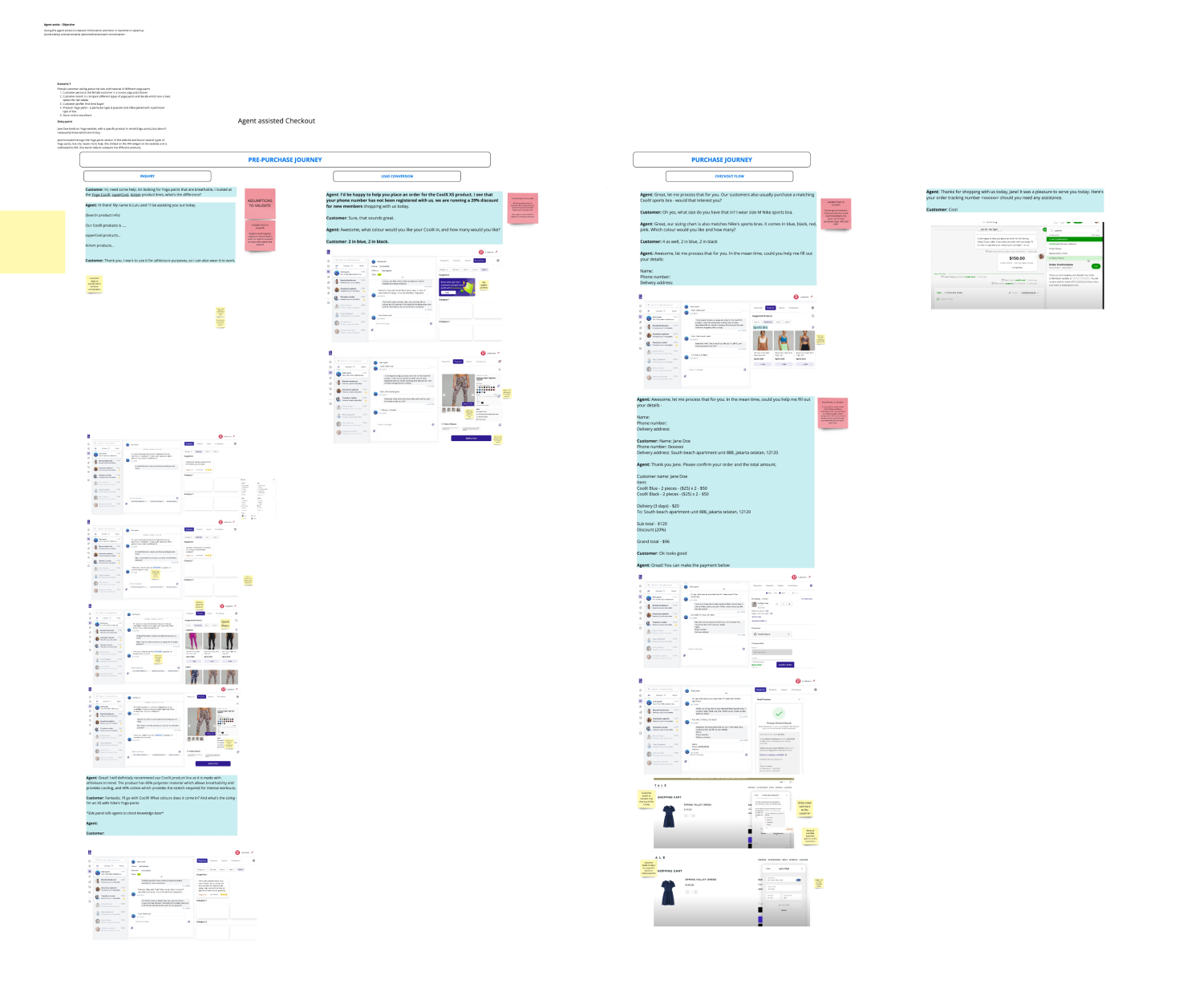
In these storyboards/scripts, it's important to identify events and situations that support the motivations behind someone's behavior.
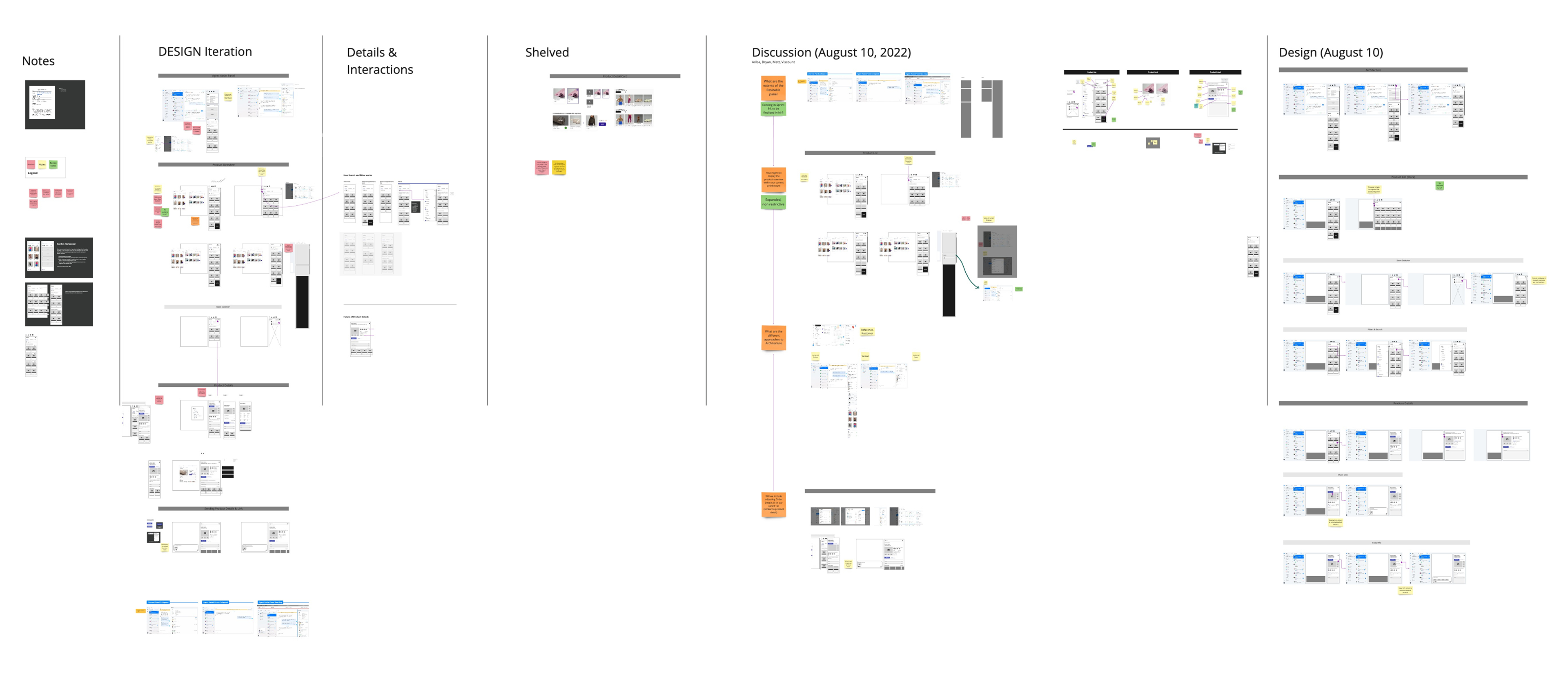
The convictions work board for Agent Assist, sorted according to the sales funnel
C - Multi-Variant Testing
We brainstormed, designed, and simultaneously tested multiple design variations in a robust three week Multi-Variant Testing (MVT) activity. Imagine a more active version of A/B testing. Creating different combinations of design elements, layouts, and functionalities (according to our MVT group's focus) and engaged with users across various organizational levels, ranging from front-line employees to CEOs throughout 15 diverse e-commerce verticals in SEA, Africa, India, and the Middle East regions.
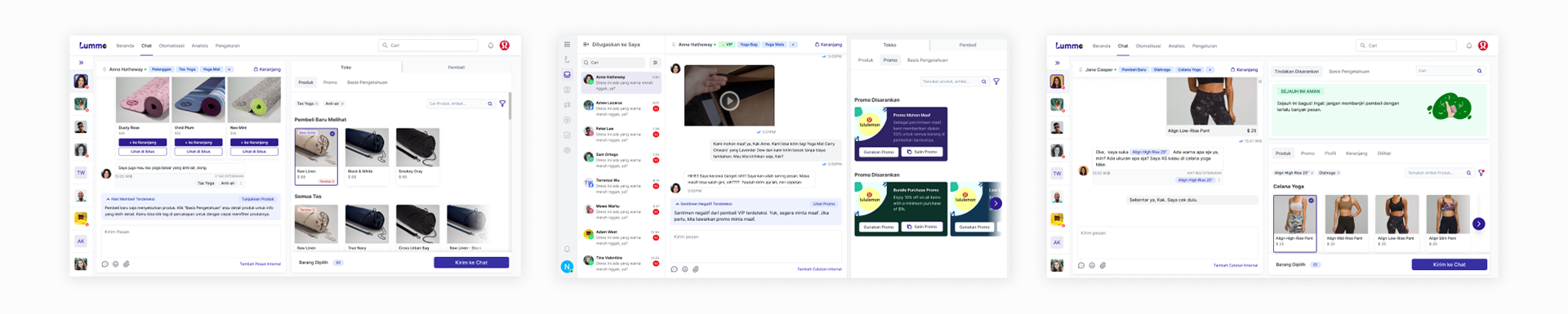
A screen from each of the three design prototyped and tested for the Agent Assist MVT group.
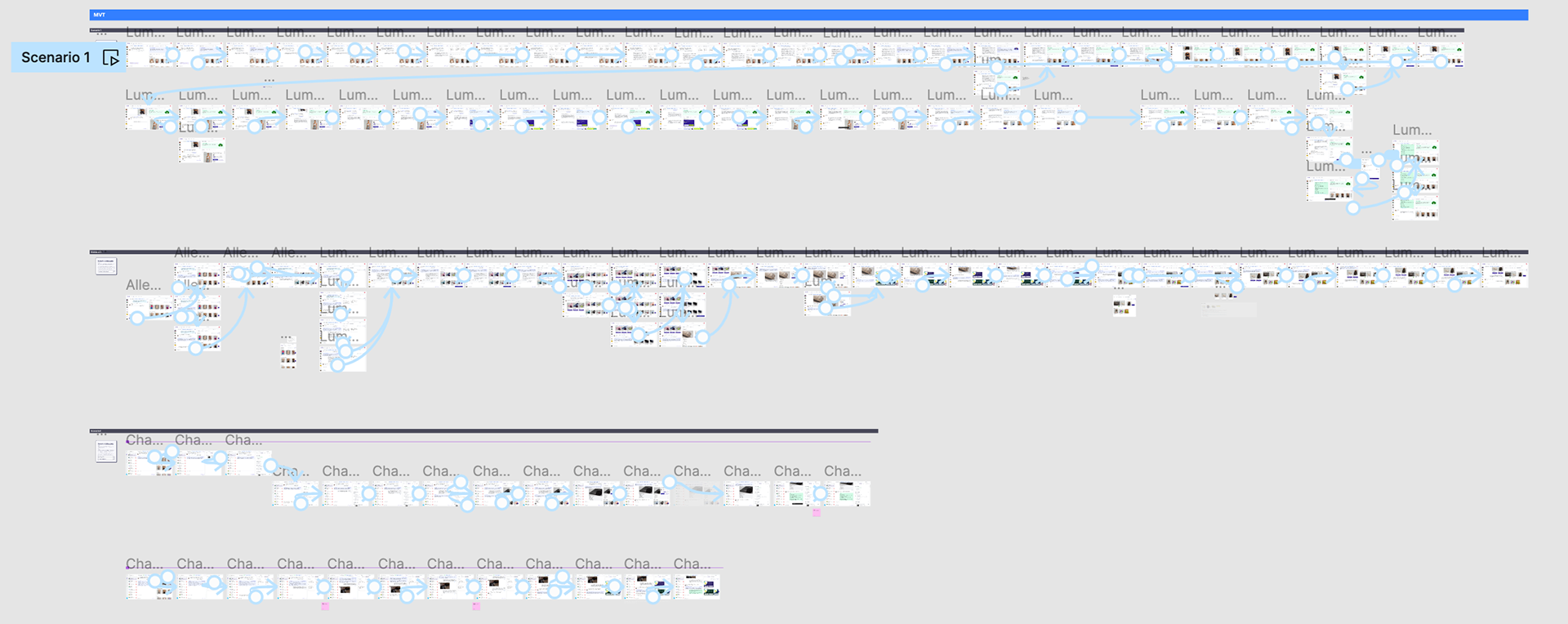
A snapshot of the three prototypes designed for the Agent Assist MVT
Through this rigorous experimentation we captured the industry leaders' deep experience, providing market insights that helped further guide our product roadmap. We identified the most effective solutions that resonated with users and aligned with our business goals.
The MVT provided strong qualitative and quantitative evidence, enabling us to make data-driven decisions.
D - Synthesis / Research Results
Collaboration and communication were at the heart of this step. Constantly iterating on designs, brainstorming, and aligning with stakeholder, engineer, and others, to formalize the product roadmap and address the subsequent technical complexities. And after uncovering the complexity and resource bandwidth, my project was broken down into two parts:
The first part, Agent Assist, focused on empowering e-commerce chat capabilities. Enabling agents to quickly access product information, leverage canned and suggested responses, and provide immediate customer assistance. This was to serve as the foundation for the next step, true automation.
The second part, Automation, are advanced features like Natural Language Processing (NLP), chatbots, multi-branched campaigns, and other conversational automations. This phase aimed to leverage cutting-edge technologies to automate and personalize customer interactions, improving overall efficiency and customer satisfaction.
Phase 2 - Design
The biggest challenge for the user experience is the user's own cognitive limit. Simply, that the amount of actions and information made available (and are necessary) for them can easily be overwhelming if not properly managed.
Through every aspect of the architecture, user experience and interface, we constantly employe multiple methods of breaking down information into manageable chunks to enhance understanding, memory retention, and communication.
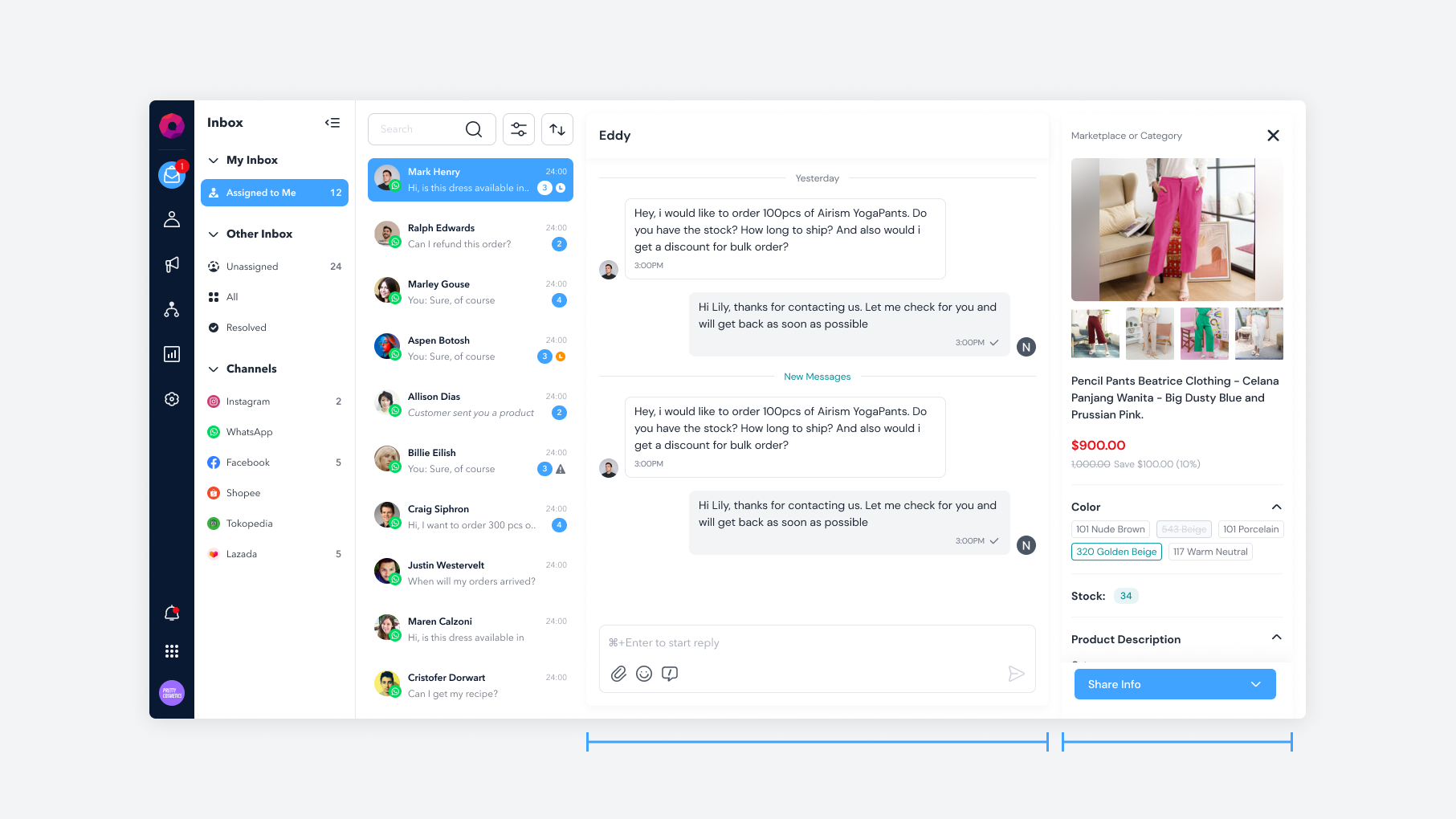
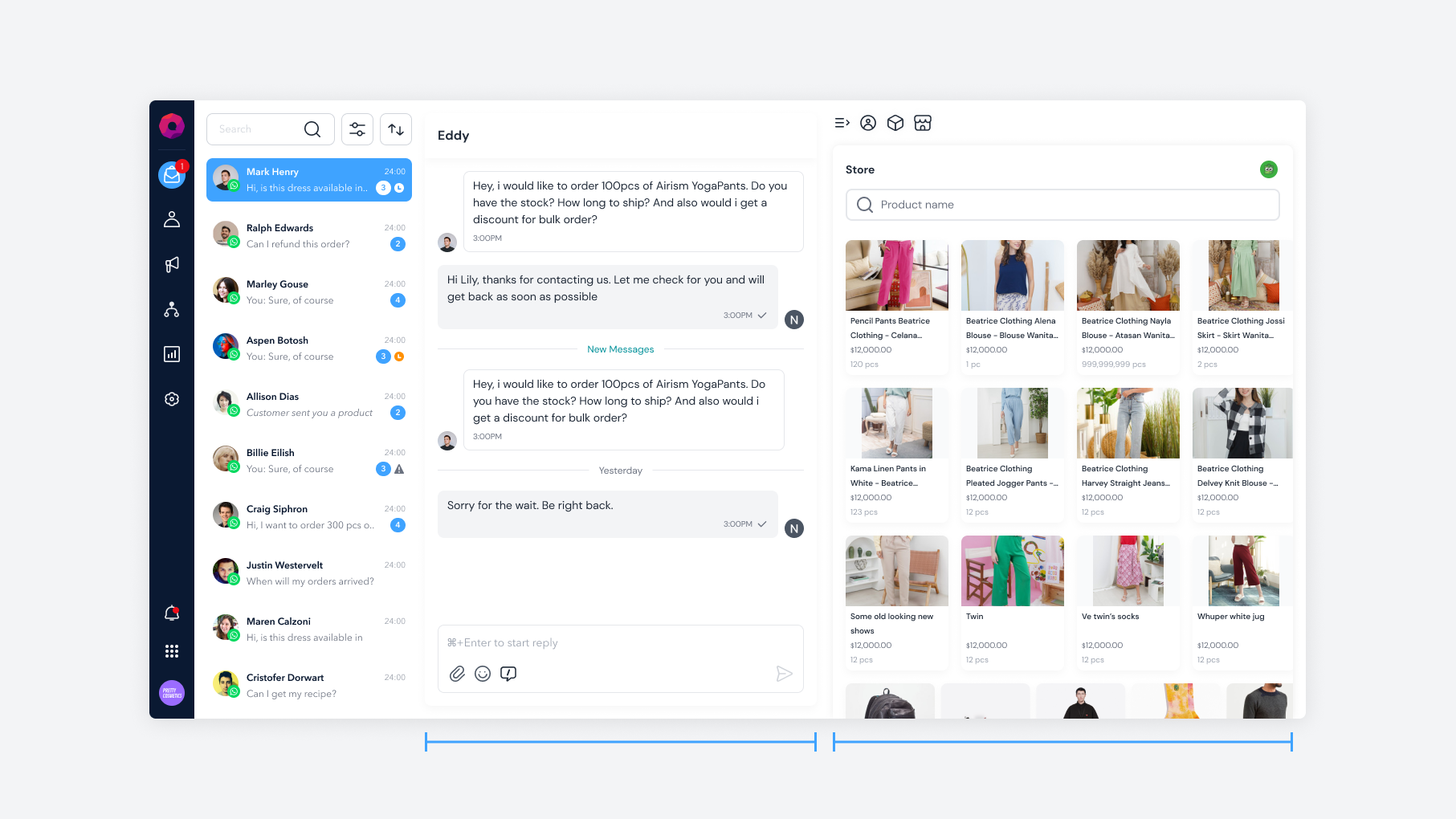
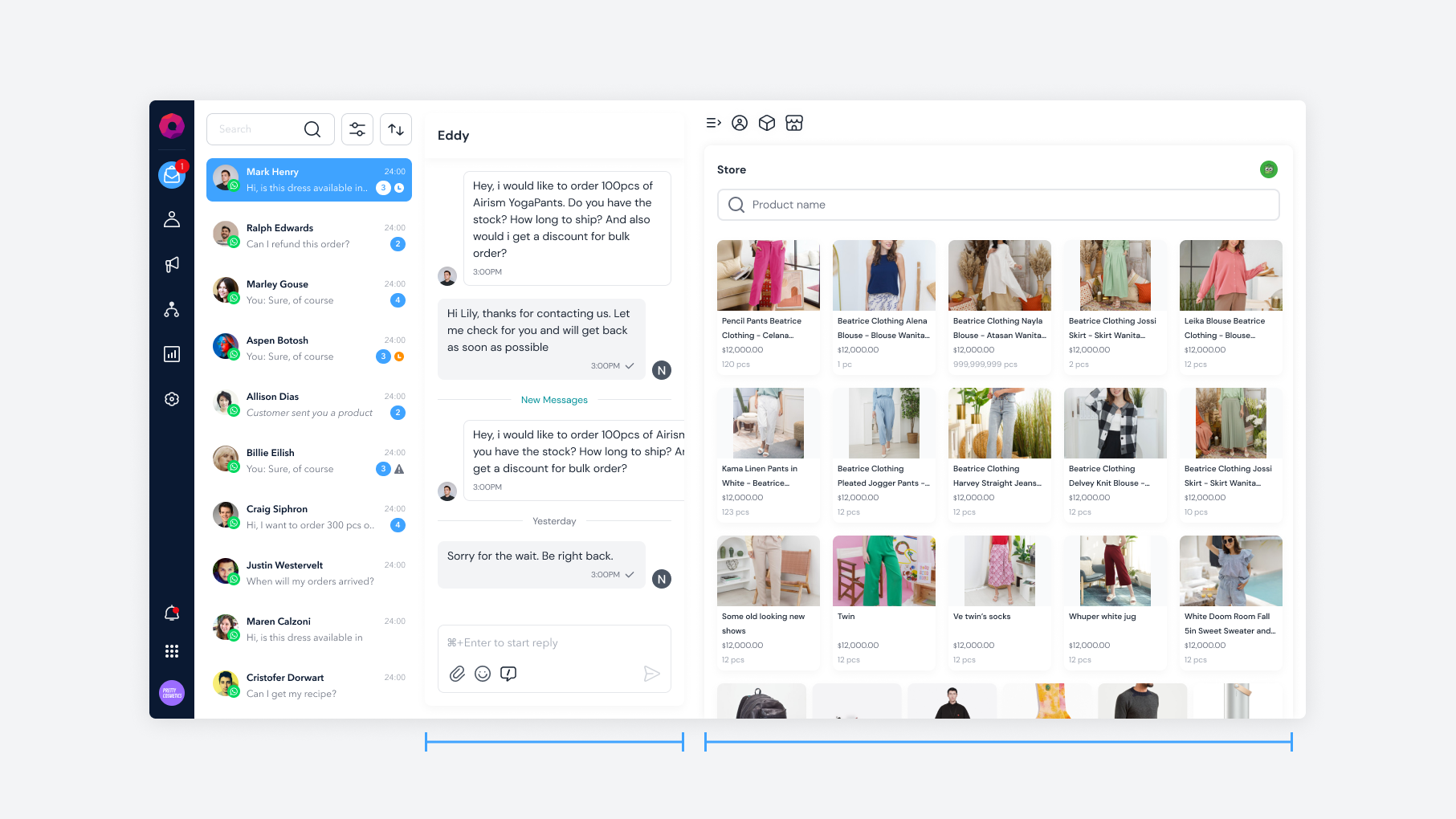
Responsive and Adaptable
The user's objectives, the features they need, tremendously vary across the day depending on the customer they're talking to. The design of the agent assist panel shifts, grows and shrinks both on-demand and reactively, maximizing the ease of use, reducing screen congestion resulting in a focused interface that enhances usability and user interaction (time to discover, and time to click), ensuring users are never encumbered by limited real estate.
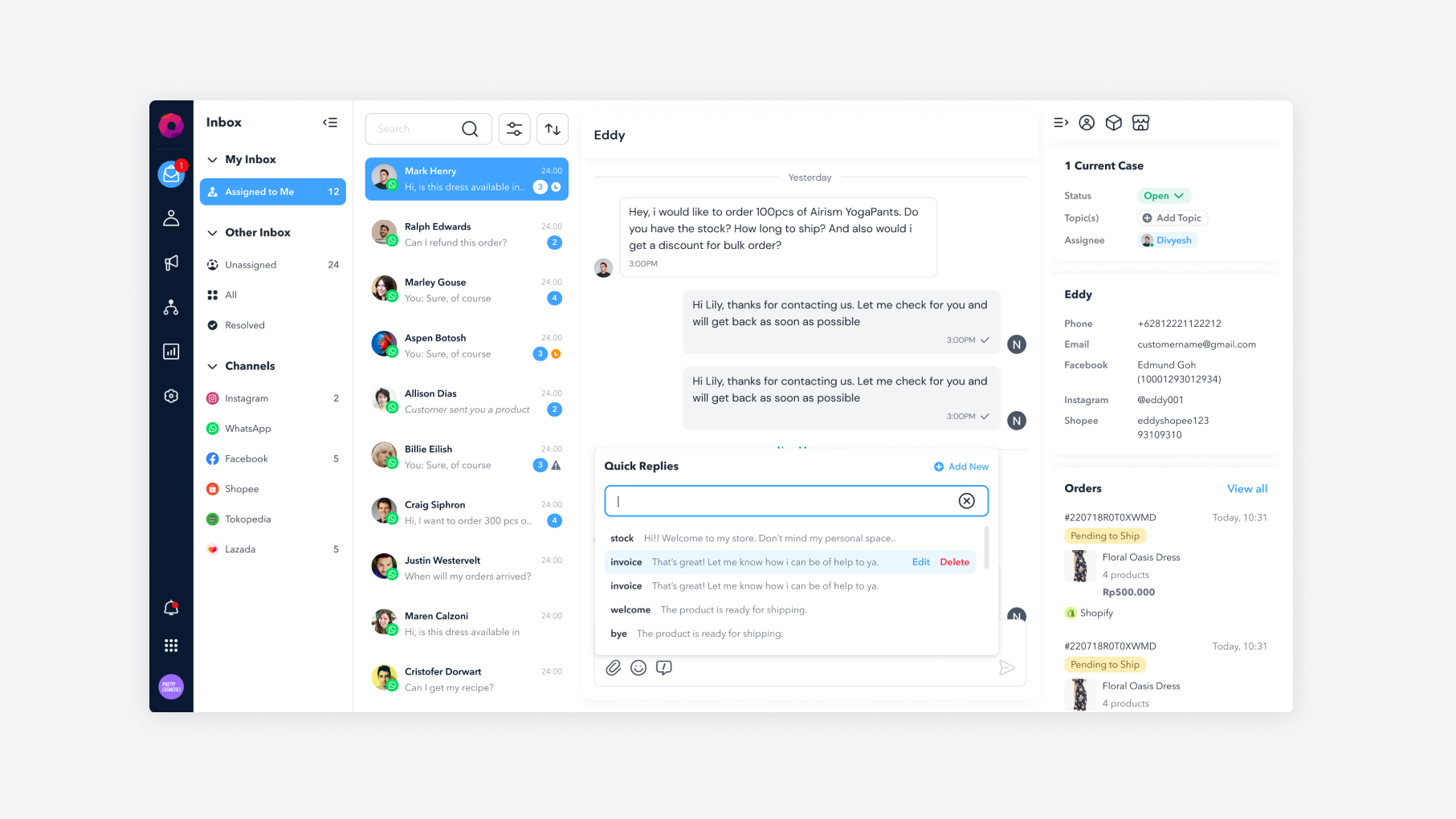

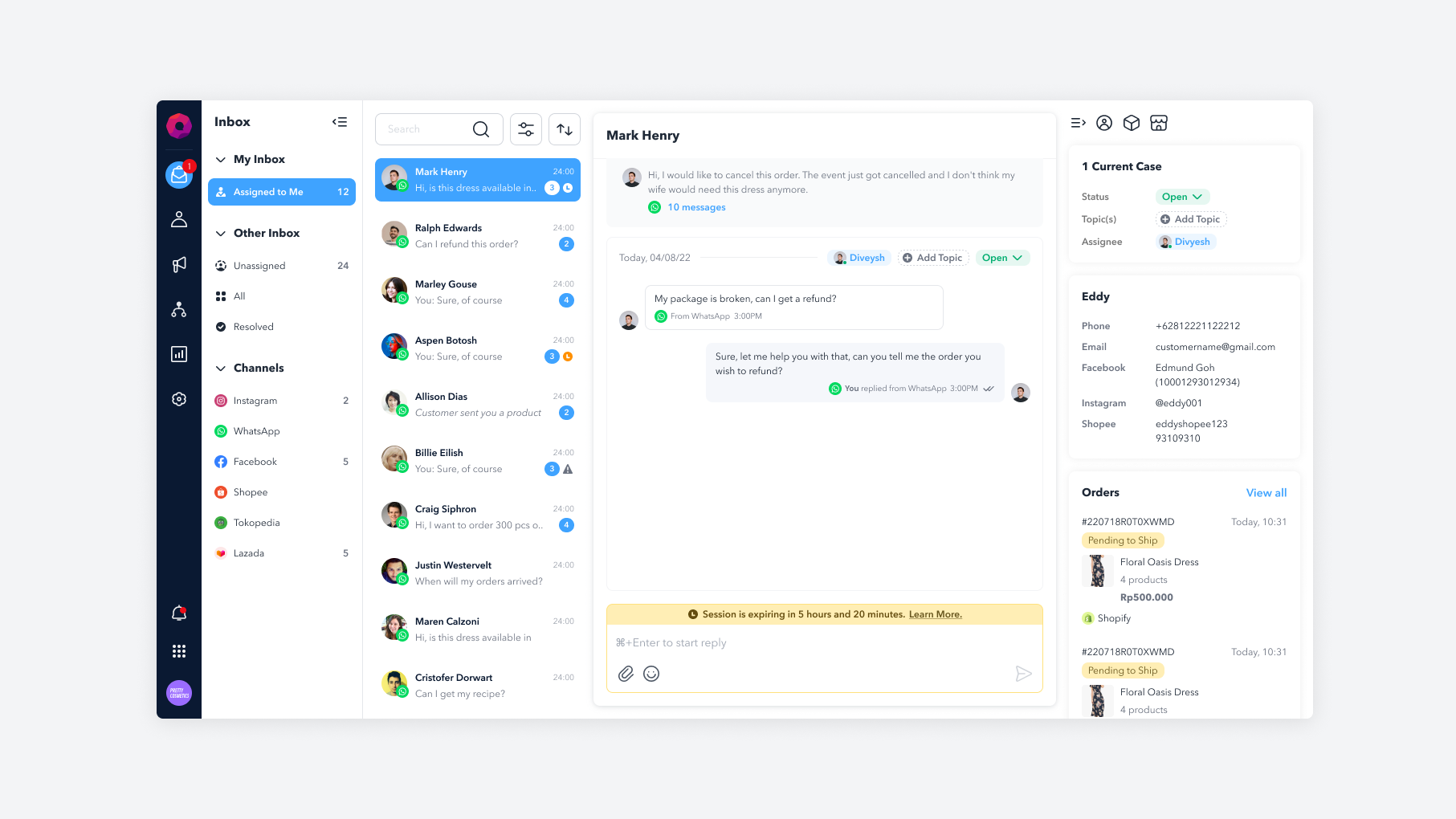
Speed and Efficiency
Backed by heavy research we were able to discover and address major needs and pain points of users beyond just keyboard shortcuts. Strategically prioritizing and streamlining features to ensure that the most essential functionalities (ex, quick replies, product details, etc.) are prominently accessible within the main chat component itself. Delivering 80% of the value with just 20% of space (Pareto Principle).
This approach allows users to quickly, and easily, access the core functionalities they need without being overwhelmed or burdened by unnecessary complexity.
Next Steps
For the design project, our next focus will be on incorporating Natural Language Processing (NLP) into all existing features. By integrating NLP across the board, we aim to create a more intelligent and responsive design that anticipates user needs and delivers a personalized experience--improving the quality of the experience for both business and consumer.
Learnings
Applying the Multi-Variant Testing process for the first time, is akin to running a gigantic Design Sprint. But at the end of it you come out with validated data, and an extremely competitive product. But more importantly, it highlights the importance of early and lasting communication (workshops, ideations, low-fi's) as it allows core information to scale along with the project and the team.
Additionally, that to succeed in this era we need a laser focus on customers, extreme operational rigor, and a burn that matches traction.
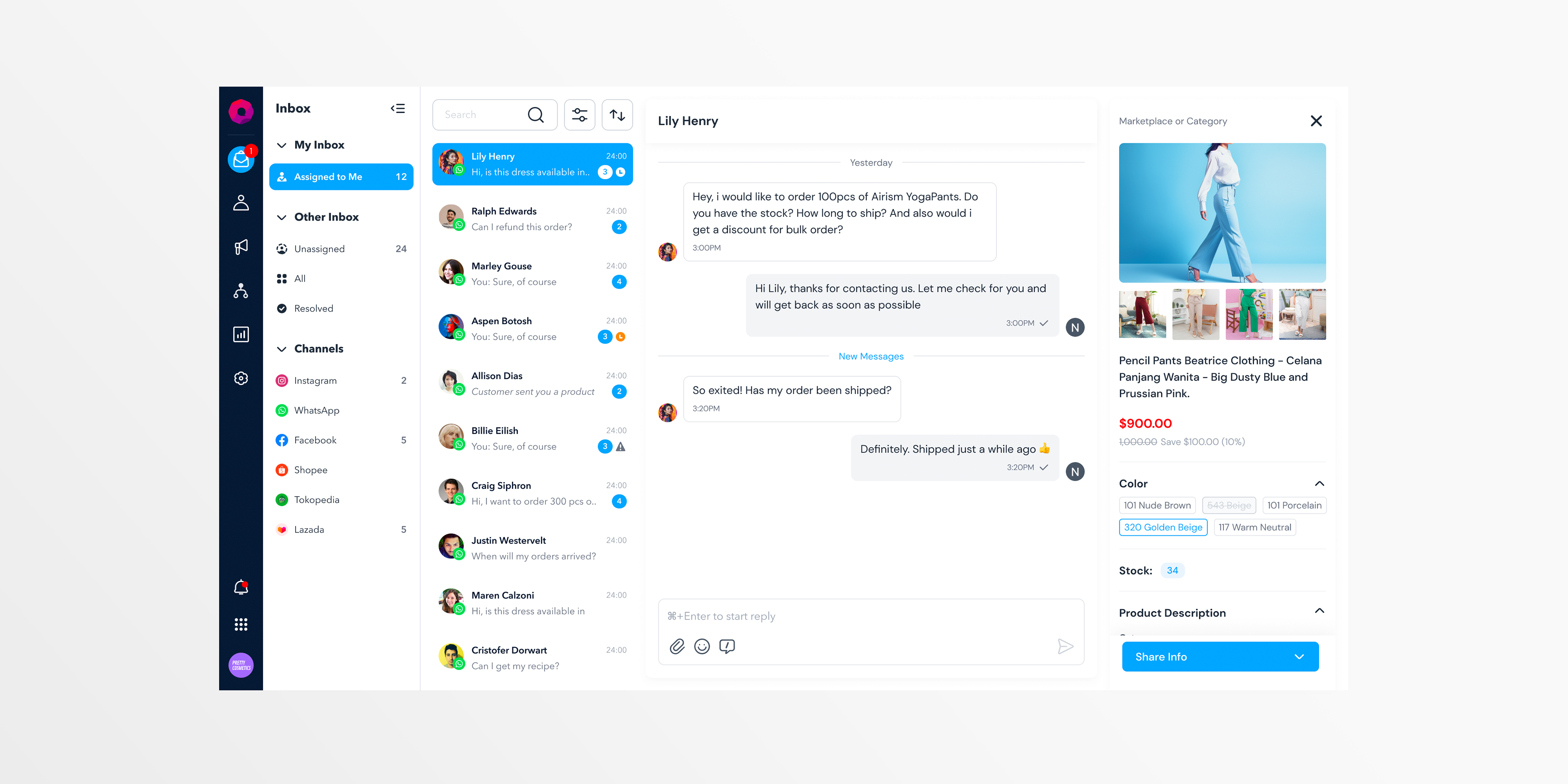
Thanks for reading!